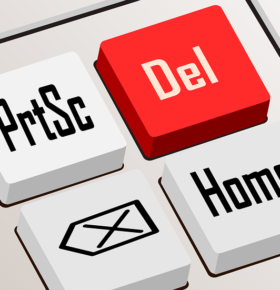
Data cleaning is probably the most important part of my job. Clean data ensures the validity of our analysis. Defining what data is considered clean, and what is not, varies from program to program and survey to survey. Determining what you will qualify as clean data, and strictly maintaining those qualifications, is paramount to ensuring the validity of your program.
Where do I start?
The first place I tend to start is determining the valid length of a survey. Surveys that take too little time generally indicate that questions were not read properly, or were otherwise neglected. To ensure the survey is long enough, we generally apply the following:
• Take the median and interquartile range of your data
• Subtract the interquartile range from your median length
This should serve as your minimum time to complete. Generally speaking, I avoid enforcing a maximum time to complete as, with online surveys, it’s always possible people start and then stop. With surveys in the field, it’s possible that the result wasn’t submitted due to network latency. Generally, just enforcing a minimum will be adequate for ensuring good data based on timing.
Another qualifier is survey completeness. Generally, we at PortMA avoid forcing consumers to answer all of the questions of the survey, as we don’t want to lose respondents just because they don’t want to answer a particular question. (e.g., if they don’t want to answer question #4, some consumers will simply drop out if they have no other option). While you can add a “Prefer not to answer” response, that’s effectively the same as letting them skip, but with no real benefit. We recommend you simply remove surveys that are not complete. We recommend something between 40% and 60% as a minimum amount of the survey completed. This ensures that you have enough responses for the result to be valid.
Finally, surveys that are missing key demographic answers should disqualify a survey. Things like market and event type are important to understand where the value is in your data. If these responses are missing, this serves as valid reasoning for removing these results. It’s important that your segments don’t differ too much from your overall.