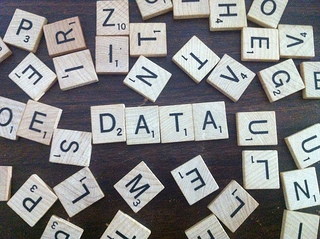
In my last post on data integrity, I spent some time talking about how to ensure quality when reviewing panel data.
In this post, I expand on that and share some tips that are useful for shorter surveys. While straight-lining can help you identify suspect results in long surveys, it doesn’t really help if your survey is less than 10 questions. As such, we will look at some other metrics you can use to define survey quality.
Know the length of time it takes to complete your survey.
This is easy to do with most online survey tools and mobile apps, as they include a start and end time function. The time between these is the survey completion time. Once you have a significant number of results, take the average of all your completion times. Then, exclude any outliers from the data (Wikipedia has an excellent page on outliers and how to calculate them). Once you have excluded all the outliers, find the standard deviation of your data. Microsoft Excel can easily do that with the =stdev function.
Anything not within two standard deviations of your average should be considered suspect and as something that might invalidate your data integrity. Those on the low end may be a result of consumers not reading questions fully. I strongly recommend removing all results that fall below this bottom limit. For those above, it takes a more instinctive call. Sometimes the results may not have been submitted immediately. That could make it appear as though it took an inordinately long time to complete the survey. Make your decisions based on informed judgment.
Focus on how much of the survey has been completed.
While this may seem like less of an issue than other problems with data integrity, it is important, nonetheless. I recommend defining certain portions of the survey that must be completed in order for the survey to be considered valid. Remove from consideration any results that do not meet this standard. How much you expect to be completed is up to you. Even 50% of a survey can be enough to ensure data quality without being entirely dependent on the number of responses you receive.
For online surveys, it is possible to require an answer for each question. This ensures that no questions are skipped. The major drawback to this is that some consumers may drop out of your survey rather than complete it. This may happen if the survey is too long or if there is a question they do not wish to answer. If you feel that it is important that every question be answered, you should set a standard of 100% completion as part of your data cleaning process. This allows you to maintain that level of integrity. Should you change your mind, you have a backup collection of data without having to go back into the field again.
There are a lot of ways to define how you are going to control your data quality. The most important is to remain consistent in how you clean the data. Set a standard methodology for data integrity at the beginning of a project and adhere to it for the duration. If you must revisit your process, be sure to apply modifications retroactively and to the entire data set.
Photo Source: https://www.flickr.com/photos/notbrucelee/to