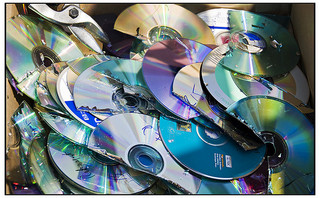
A previous post outlined key considerations for designing a “perfect” survey and described the impact survey design can have on data quality. But ensuring actionable data is not guaranteed simply by deploying the perfect survey.
The next step to ensuring great market research data involves cleaning your data prior to analysis. While not an exhaustive list, the four areas below offer some ways to ensure the quality and integrity of your data are protected and maintained prior to analysis.
Identify a threshold for flagging bad market research data
Despite being asked beautifully worded questions, respondents still may provide poor quality responses. One way to determine respondent quality is to look at the length of time to complete the survey and patterns of straight-lining.
For example, suppose a handful of respondents completed a survey in one minute or less when it should have taken five. Chances are, they blew through the survey without reading it thoroughly (presuming there are no skip patterns that allowed them to answer just a few questions).
In addition, if it appears they clicked on the first response for every question, or gave every item in a grid the same rating, you may want to consider whether you want them in your data set.
Account for missing market research data
Sometimes, survey questions are not required, giving respondents the option to answer a question rather than force them to do so. This approach can contribute to a better respondent experience and helps minimize untrue responses.
However, if you have missing data it is advisable to assess the percentage and distribution of missing data and determine what impact, if any, missing data will have on the statistical analysis to be used. Missing data can violate the assumptions of certain advanced analyses, which essentially means you run the risk of reaching an incorrect conclusion based on your analysis of the data.
Abstain from cherry picking
Cherry picking, or discarding unfavorable responses, is a form of data manipulation, a cardinal sin (perhaps the cardinal sin) in the world of research.
Seemingly negative responses are still valid, and while they may not paint a pretty picture, they do offer the opportunity to learn something from respondents. (For more on addressing concerns around unfavorable responses, see Turning Bad News Into An Opportunity.)
Make sure market research data flow is correct
Run a set of frequencies on all questions to make sure that response patterns account for any programming logic used in the survey (e.g., ensure questions that were supposed to be asked only of female respondents were not answered by males).
Similarly, reviewing basic counts allows you to look at the distribution of your market research data, which helps make sure you select the correct analysis to use, and perhaps more practically, affords greater insight into the value of your survey.
For example, if you asked respondents to rate a product using a series of attributes, and found out that 90% of all responses were rated a 10 on a 10 point scale, how valuable and/ or actionable is that data? Do you need to adjust the attribute list or ask a different question altogether? What steps do you take to ensure data quality is protected?
Photo Source: https://www.flickr.com/photos/swanksalot/