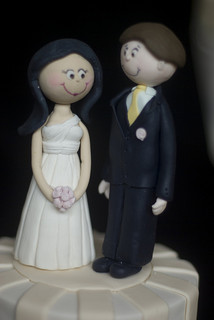
Receiving information from multiple sources can be both a blessing and curse. Multiple sources lets you get data faster, and be more confident you will hit your desired sample size, something clearly pivotal to research. That being said, it is important to be careful when working with multiple sources, as a number of ill effects can come of it.
1. Remove repeat respondents from the data
When looking at research that comes from multiple sources, the first priority is to make sure your research included a question to make sure that the same consumer didn’t take your survey in multiple occasions.
A simple “have you taken any surveys related to X in the past week” is usually adequate enough to cover you. You may generate false positives occasionally, but the added security of not double-sampling is worth that risk.
2. Check for demographic differences in your data sets
Next, you need to compare your disparate sources.
Line them up item-by-item and check for significant differences. Any significant differences indicate that your sampling pools were not the same.
Small differences about one item can likely be explained away easily enough, but if the data is systemically different, you need to begin looking for the cause(s) of the differences.
Begin with your demographic data. One source sampling 20% more women could easily cause disparity within your data, despite your total populations being similar.
As you find areas that may explain the variation, compare their significance while filtering for each of those components. If you are finding your differences are generally disappearing when filtering, then that likely explains the difference in your overall data set.
3. Check for psychographic and other differences in your data sets
If you cannot find anything that explains your differences based on demographics, it is likely that your data is different, and it is now up to you to leverage that in a proactive way. This requires looking at what is different between your two groups’ results, and attempting to infer what difference is causing this change.
A much lower emphasis on price could indicate that one group has a higher overall income, or a different overall geographic spread, causing them to have different impressions of a product based on the advertising they have seen.
This type of inference will determine much of how you view the data, but it is important never to be confident you are 100% correct, and to base your conclusions on what you know, rather than what you believe.
Photo Source: https://www.flickr.com/photos/mags_eb/