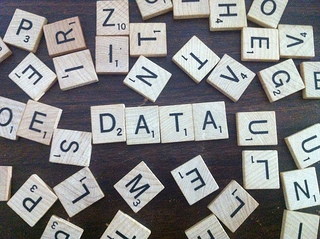
Modern companies have access to huge volumes of user data that they can analyze to provide more personalized services. Data is not just big business, it is a seminal weapon in the fulfillment of business strategies.
Understanding which aspects of user behavior are statistically significant, or are likely to influence other aspects, is crucial when charting the path for your company. In this article, we will explore the importance of causality in data analysis and what steps you can take to find causal links within your own business data.
Estimated reading time: 4 minutes
What is Causality and Why Does it Matter?
The accurate analysis of data requires a firm understanding of causal links, used to optimize your resources on the most critical aspects of your business. This is where causality comes in. While it’s easy to focus on the data you want to monitor, remember that what happens before and after an event can provide valuable insight into what happened. This will help you devise better questions for analysis while also providing new KPIs that may otherwise be lost in the data.
Control Variable Analysis
There will naturally be some variables you have control over, such as the days of the week and times you can run certain marketing campaigns. It’s these causal things that you are putting together that you believe are going to have an outcome; the things that you have control over. Once you identify them, you want to bring in the data that represents the outcome. It could be sales volume, click-throughs, event impressions, etc. Then, you want to have metrics around the effect, which is what you’re trying to measure the impact of.
When referring to controlled variables, they often involve things that are categorical. Categorical variables have categories as opposed to a specific order. For example, venues for an event could be street intercept, an affair festival, a concert, or a retail activation. With controls being categorical, we want to measure them. Your outcomes should be more like a ratio or ordinal variable, which is something that can go from zero to infinity or has some kind of order like small, medium, and large.
(You can listen to the full episode of the podcast below.)
How Do You Analyze Data?
Once you have the data, determine how you’re going to analyze it. First, you need to look at the frequency of your measures. There are multiple ways to do this, like using a histogram or averages, that are helpful to understand the data. Through this, you’ll get the rate of occurrence of your variables. The purpose is to segment the outcomes that can help you identify which areas are higher than average and which are lower. You can then control for the variables that make your categories high or low.
Minimum Requirements
Note that when comparing occurrences of your outcome among segments or causal variables, you need at least 30 occurrences per category before you detect any significant differences. This applies when doing statistical tests to make sure that there are no other factors in the data that might mislead you. In larger cases, it can be a good idea to have a sum of 400 to allow for the generalization of a large population. Cases less than these numbers are more prone to skewed data.
For example, if you’re considering how often you’re reaching men at on-premise activations versus retail store, or sell off, you’re meeting women at on-premise activations versus retail, if a difference you find is 10 points or more, then it’s a good idea to lean toward that idea of this being a real significant difference. A larger population size of 400 will help you identify which differences are significant and which may be biased or not true.
Using Causality Effectively
Causality is the cornerstone of data analysis. By identifying causal links, you can use this to predict future outcomes. Identify the potential variables that lead to success or failure and create KPIs based on these findings. And remember, stop guessing at causality through random observations – only by analyzing your data properly will you see what’s really driving your business forward.
If you missed Part One of Making Better Decisions with Data, it can be found here.
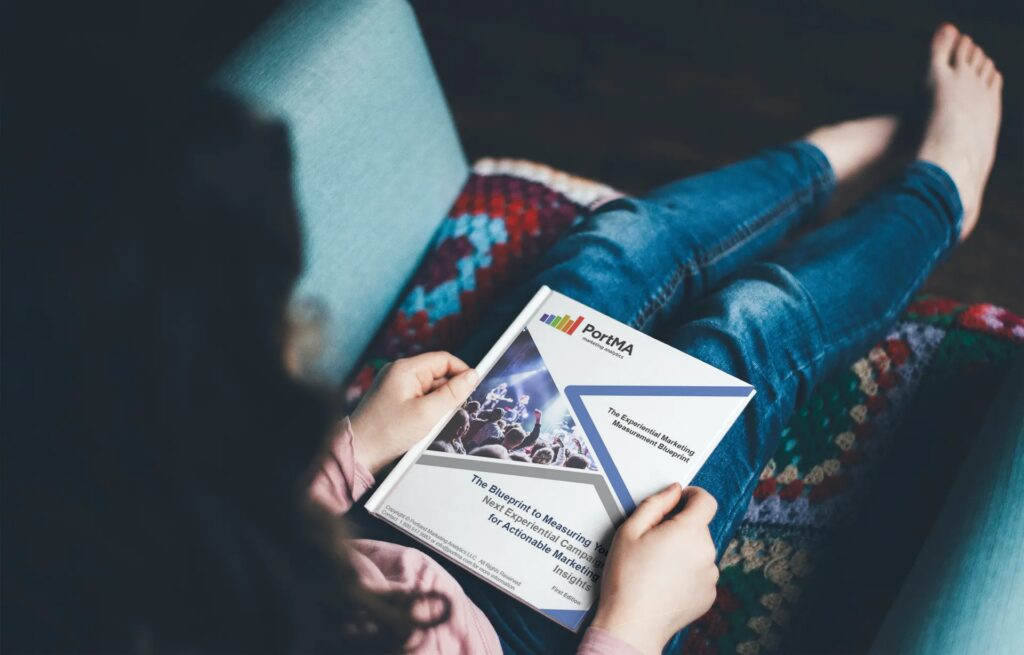
Additional Resources
FOR EXPERIENTIAL MARKETERS
- Experiential Measurement Blueprint
- Event Impression Calculator
- Experiential ROI Benchmarking Reports
- Event Measurement Video Tutorials